Exyte provides efficient design and construction solutions
Exyte delivers comprehensive data center services from design to construction, ensuring efficiency and reliability. With our integrated project delivery approach, we have successfully executed numerous projects worldwide, including advanced intelligence data centers, hyperscale data centers, colocation and cloud-computing data centers using traditional construction as well as modular construction techniques.
Exyte has over two decades of experience in delivering industry-leading data centers with innovative solutions to meet today's advanced computing technology needs. Among our clients are some of the most demanding data center providers, renowned for their stringent requirements for reliability, efficiency, and sustainability. All of this positions Exyte at the forefront of innovation in the data center market space.
Our expertise spans all aspects of data center facilities, focusing on utilizing the most current, proven energy delivery technologies through reliable commissioning practices which allows Exyte to accelerate our client's speed to market. As a provider of efficient and sustainable data center solutions, Exyte contributes to the global megatrends of digitalization and connectivity, e-mobility, sustainable energy, and digital health.
Introducing Exyte's global data center experience
Our clients benefit from over twenty years of global data center experience, tailored to specific strategy and project requirements. Design and front-end engineering, delivery, commissioning, or ongoing facility performance optimization, Exyte Provides local delivery solutions based on our global experience to meet the needs of our clients.
Value chain expertise
From engineering and design to commissioning, Exyte offers end-to-end services to streamline every data center project. Our key strengths lie in leveraging cutting-edge technical knowledge for project success, ensuring fast project kick-off and execution with our standardized integrated delivery process and contract flexibility, and staying within budget for maximum return on investment with robust market data and cost intelligence.
Exyte has over two decades of experience in delivering industry-leading data centers with innovative solutions to meet today's advanced computing technology needs. Among our clients are some of the most demanding data center providers, renowned for their stringent requirements for reliability, efficiency, and sustainability. All of this positions Exyte at the forefront of innovation in the data center market space.
Our expertise spans all aspects of data center facilities, focusing on utilizing the most current, proven energy delivery technologies through reliable commissioning practices which allows Exyte to accelerate our client's speed to market. As a provider of efficient and sustainable data center solutions, Exyte contributes to the global megatrends of digitalization and connectivity, e-mobility, sustainable energy, and digital health.
Introducing Exyte's global data center experience
Our clients benefit from over twenty years of global data center experience, tailored to specific strategy and project requirements. Design and front-end engineering, delivery, commissioning, or ongoing facility performance optimization, Exyte Provides local delivery solutions based on our global experience to meet the needs of our clients.
Value chain expertise
From engineering and design to commissioning, Exyte offers end-to-end services to streamline every data center project. Our key strengths lie in leveraging cutting-edge technical knowledge for project success, ensuring fast project kick-off and execution with our standardized integrated delivery process and contract flexibility, and staying within budget for maximum return on investment with robust market data and cost intelligence.
Locations
With its headquarters in Stuttgart, Germany, and offices worldwide, Exyte continues to lead our industry globally. Find the location nearest to you for more information.
.jpeg?w=3840)
Americas Region (AMER)
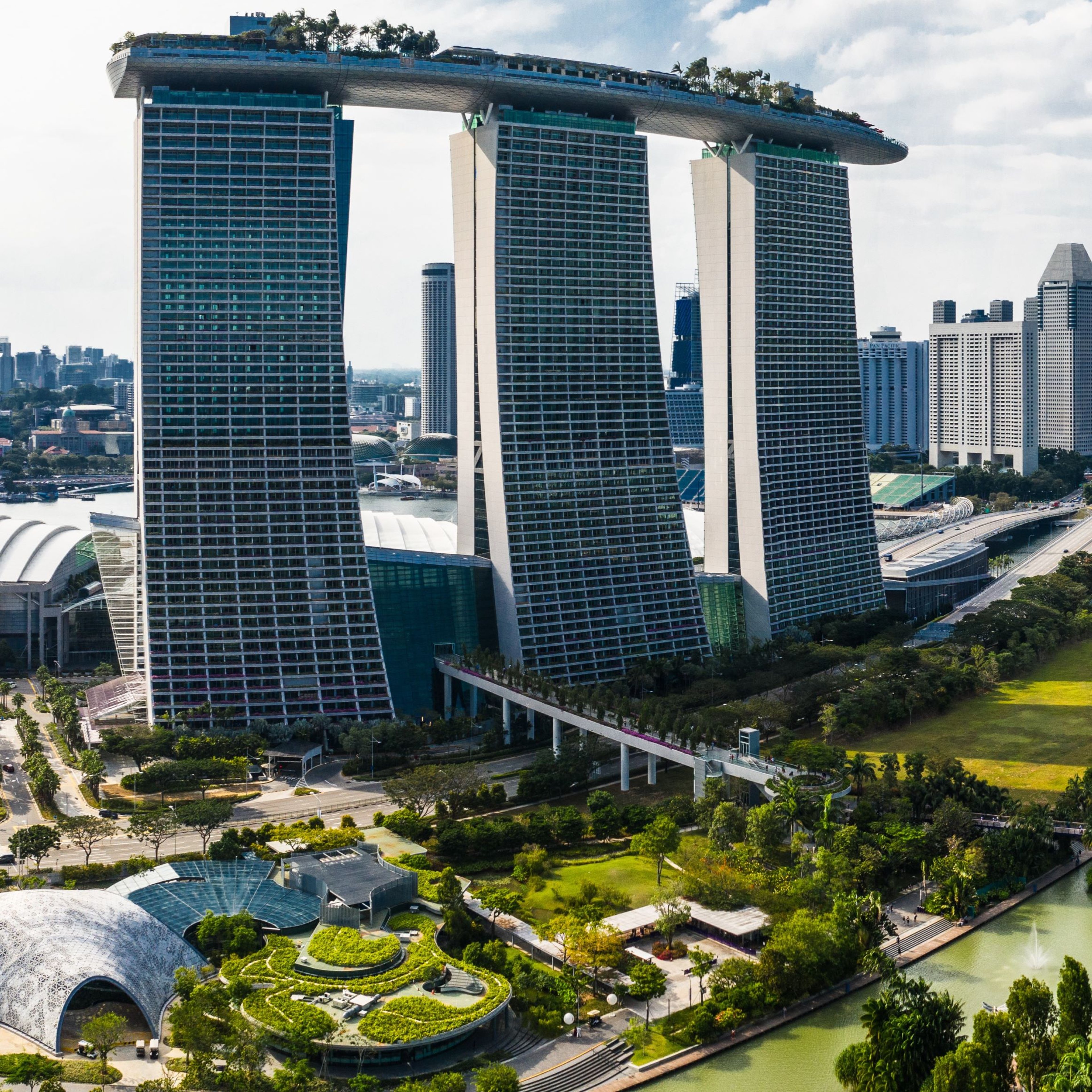
Asia-Pacific Region (APAC)
.jpeg?w=3840)
Europe Region (EMEA)
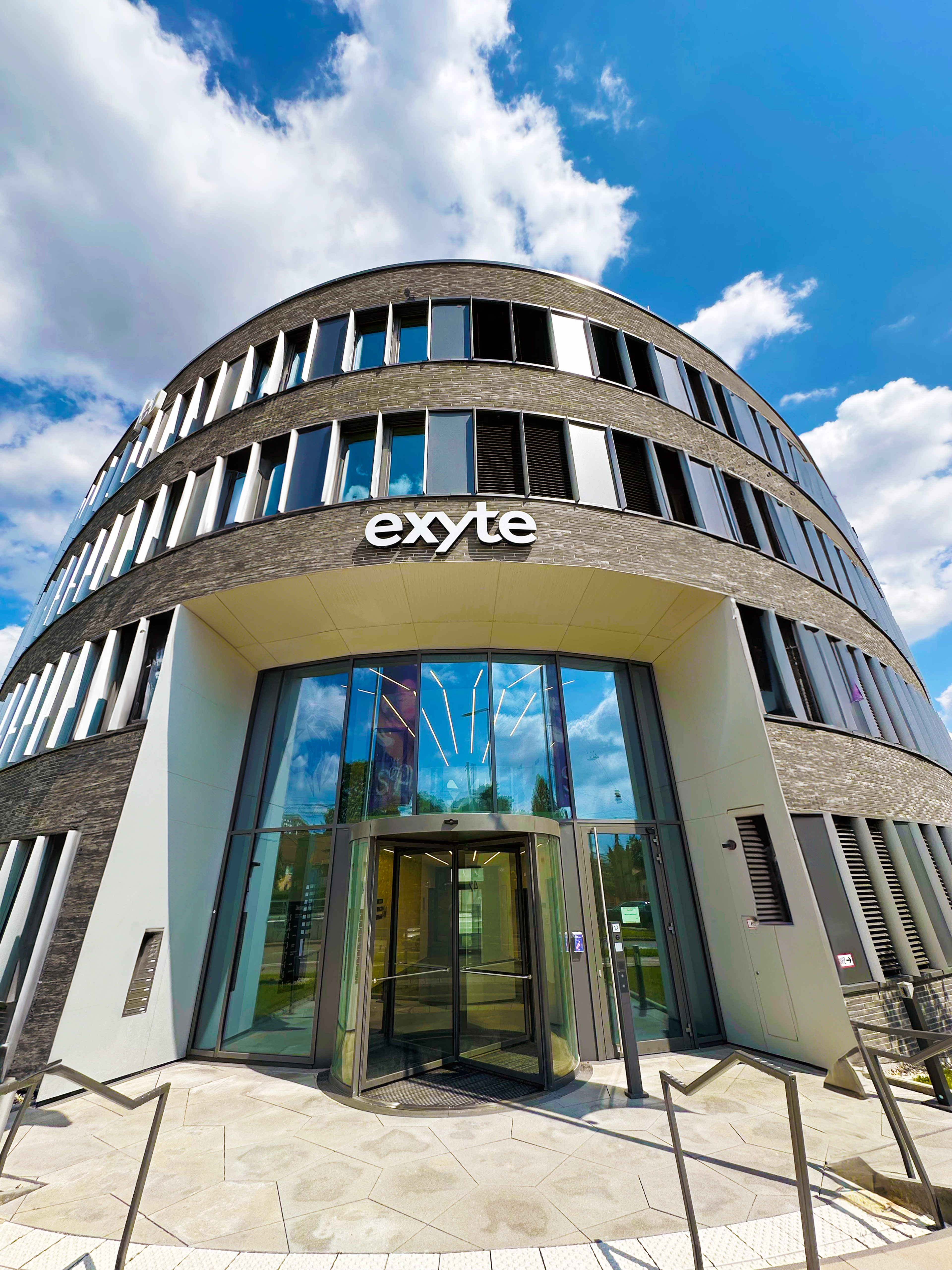